Anomaly Detection
Network anomaly detection is a critical element in modern cybersecurity strategies, providing organizations with the means to proactively identify and mitigate potential threats to their network infrastructure.
Building Network Shields: Securing Cyberspace Through Anomaly Detection
Network anomaly detection is a crucial aspect of cybersecurity that involves identifying abnormal patterns or behaviors within a computer network. The primary goal is to detect suspicious activities that deviate from normal network behavior, which may indicate potential security threats or malicious activities.
Anomaly detection is applied across various network components, including intrusion detection systems (IDS), firewalls, and endpoint security. It helps in identifying unauthorized access, malware infections, data exfiltration, and other cyber threats.
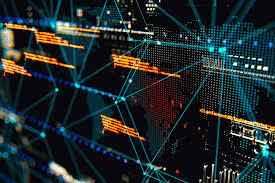
Detecting anomalies in a network is a critical facet of cybersecurity, involving the identification of irregular behavior or patterns within a computer network. The primary objective is to pinpoint activities that deviate from the expected norm, signaling potential security threats or malicious actions. Anomaly detection is pivotal for bolstering the overall security stance of a network by facilitating prompt responses to potential threats.
Types of Anomalies:
Point Anomalies: Single instances of data that deviate from the norm.
Contextual Anomalies: Deviations based on specific contexts or conditions.
Collective Anomalies: A set of data instances that collectively deviate from normal behavior.
Key aspects of network anomaly detection:
Behavioral Analysis:
- Network anomaly detection relies on behavioral analysis to establish a baseline of normal network activities.
- This baseline, drawn from historical data, encapsulates the expected behavior of users, devices, and applications within the network.
- Deviations from this baseline are flagged as anomalies, which may indicate issues like unauthorized access, malware infections, or other security incidents.
Machine Learning and AI:
- Machine learning and artificial intelligence (AI) play a significant role in network anomaly detection.
- These advanced technologies can analyze substantial volumes of data and automatically identify patterns or anomalies that might pose challenges for traditional rule-based systems.
- Commonly employed approaches include supervised learning models trained on labeled datasets and unsupervised learning models that identify anomalies without prior training.
- Employ supervised or unsupervised learning algorithms to recognize patterns and anomalies.
Signature-Based Detection:
- Signature-based detection entails comparing network traffic against a database of known attack signatures.
- When a match is found, the system identifies the activity as malicious.
- While effective against known threats, this approach may struggle with novel or previously unseen attack methods.
Flow Analysis:
- Flow analysis involves monitoring the flow of data between devices on a network.
- Anomalies can be detected by scrutinizing deviations from normal traffic patterns, such as unexpected spikes in data volume, unusual communication patterns, or irregular data transfers.
Statistical Methods:
- Statistical methods are employed to detect anomalies based on deviations from statistical norms.
- Measures like mean, median, standard deviation, and more are utilized.
- Unusual values or patterns falling outside expected statistical bounds may be flagged for further investigation.
- Utilize statistical models to identify outliers or deviations from the norm.
Real-Time Monitoring:
- An effective anomaly detection system operates in real-time, issuing instant alerts upon detecting suspicious activities.
- Real-time monitoring enables swift responses to security incidents, minimizing potential damage.
Normal Behavior Modeling:
- Anomaly detection relies on understanding what constitutes normal behavior within a network. This involves establishing a baseline by analyzing historical network traffic, protocols, and user activities during typical operation periods. Machine learning algorithms are often employed to model and learn these normal patterns.
- Behavioral Analysis: Network anomaly detection relies on behavioral analysis to establish a baseline of normal network activities. This baseline, drawn from historical data, encapsulates the expected behavior of users, devices, and applications within the network. Deviations from this baseline are flagged as anomalies, which may indicate issues like unauthorized access, malware infections, or other security incidents.
Integration with Security Information and Event Management (SIEM):
- Network anomaly detection is often integrated with Security Information and Event Management (SIEM) systems to provide a comprehensive view of security events.
- SIEM platforms collect and correlate data from various sources, including anomaly detection systems, offering a centralized and holistic approach to network security.
Challenges to Network Anomaly Detection
- Network anomaly detection faces challenges such as false positives (misidentifying normal behavior as anomalous) and false negatives (failing to detect actual anomalies).
- Striking a balance between precision and recall is crucial to avoid overwhelming security teams with false alerts or missing genuine threats.
- False Positives: Anomaly detection systems may generate false positives, flagging normal behavior as suspicious. Striking the right balance between sensitivity and specificity is crucial.
- Adaptability: Networks evolve, and normal behavior may change over time. Anomaly detection systems need to adapt and learn continuously to avoid becoming obsolete.
- Integration with Security Operations: Anomaly detection is typically integrated into a broader security operations framework. When anomalies are detected, the system may trigger alerts, initiate incident response procedures, or even automatically implement predefined security measures.
- Network anomaly detection is a critical element in modern cybersecurity strategies, providing organizations with the means to proactively identify and mitigate potential threats to their network infrastructure. As technology advances, continuous refinement and innovation in anomaly detection methods will be essential to stay ahead of evolving cyber threats.
- Anomaly detection plays a vital role in enhancing the overall security posture of an organization by providing early warnings and allowing prompt responses to potential cyber threats.
- As cyber threats become more sophisticated, the integration of artificial intelligence (AI) and machine learning (ML) in anomaly detection is likely to increase. The use of behavioral analytics and threat intelligence feeds will further enhance the accuracy and effectiveness of anomaly detection systems.
Network anomaly detection stands as a vital component of modern cybersecurity strategies. By harnessing advanced technologies and methodologies, organizations can proactively identify and respond to potential security threats, thereby safeguarding their networks and data from malicious activities.
Let's Secure Your Network with Anomaly Detection Strategies!
Reach out to Redline Networks to help your organization with network anomaly detection solutions